Artificial intelligence in scientific research: Common problems and potential solutions
Author(s):
Connor Nurmi
Momoko Ueda, PhD
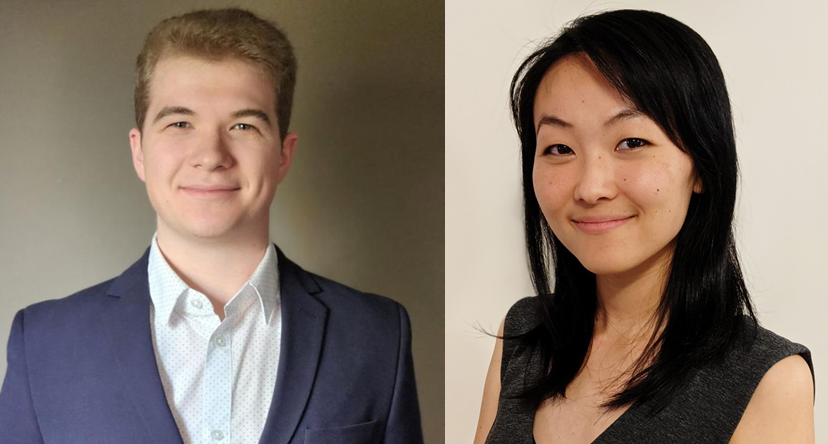
Disclaimer: The French version of this editorial has been auto-translated and has not been approved by the author.
The recent emergence of large language model (LLM)-based chatbots like Open AI’s ChatGPT have ignited the public’s interest in artificial intelligence (AI). For the average AI enthusiast, LLM-based chatbots are a convenient tool that can be easily used to complete simple tasks such as writing mundane emails, explaining difficult topics, and proofreading documents.
For scientists however, new AI tools have been developed, including LLMs that can analyze large and complex datasets, identify patterns, and extract useful information, with the ultimate goal of improving the efficiency of research. One such example is an LLM tool called Elicit, developed by AI scientists Jungwon Byun and Andreas Stuhlmüller, which functions to automatically extract key information from scientific studies like sample population size, metrics, and experimental outcomes. While these tools serve to help scientists save time and improve research outcomes, there are many issues that must be addressed and more strict regulatory policies that need to be developed before AI can be fully integrated within the research community.
In general, AI, and LLMs in particular, may face imminent challenges when training new generative models. One significant problem concerns the quality of data used to train these models, where attempts to improve datasets can limit its effectiveness. Take ChatGPT, for example; it already incorporates an extensive amount of publicly available human-generated textual data to generate answers to queries. However, if one were to scale such a model to try and improve its effectiveness, a viable solution to augment the training dataset may be to employ synthetic, or artificially generated, data. However, this approach unintentionally gives rise to a phenomenon known as “model collapse”, wherein the model gradually neglects and disregards less frequent information, ultimately leading to a degradation in performance. It is important for researchers who wish to improve the quality or quantity of databases used to train AI algorithms employed in studies to avoid supplementing with synthetic data. Existing databases should also be carefully inspected and verified before use to avoid unintentionally using data that was artificially generated.
Another critical consideration on the horizon for advancing language models involves utilizing human-generated text rooted in sensitive domains like patient or confidential data. This issue becomes especially pertinent when contemplating the application of LLMs in medical research, where integrating patient data could substantially enhance model capabilities. Methods on how to gain access to information on the inner-workings of the database used to train the LLM are relatively easy and could be performed by individuals with limited AI computational expertise, and could potentially be used to access confidential patient information embedded within. Attention must be paid to preserving privacy and precluding any identifiable patient information from making its way into the training dataset and researchers should take all necessary precautions to ensure the AI platform used is secure.
AI platforms used in scientific research have also been criticized because of its overuse, where traditional statistical techniques have been continuously shown to outperform novel AI platforms for the same application. For example, a 2019 review published in the Journal of Clinical Epidemiology, examined 71 studies that used machine learning (ML), a type of AI platform, to predict clinical diagnosis or outcomes of various diseases and found no evidence in superior prediction using ML methods compared to the more commonly used logistic regression modeling. A similar review in 2020 examined 15 studies that used ML to predict clinical outcomes of traumatic brain injury and again, found no benefit to using it compared to traditional statistical methods. Currently, no policies exist that explicitly compel researchers to validate the use of AI in performing statistical modeling. Thus, guidelines for the choice of statistical approach should be included in future policies that enable research to leverage the benefits of AI compared to traditional statistical techniques.
In contrast to AI overuse, misuse can occur where datasets that are poorly chosen can lead to the development of biased, and sometimes even sexist and racist, AI models. Google Translate, which uses a type of AI called natural language processing (NLP) to power its online translation tool, often defaults to masculine pronouns over feminine ones during text translations. This is because the web-based English language database used to train the NLP-powered machine translation algorithm contained twice as many masculine pronouns to feminine pronouns. In addition, each masculine pronoun that was output by the program fed into the database used to train the translation algorithm, increasing the problem via positive feedback. In a scientific context, poor quality data used to train new AI models can lead to similar situations, where bias can be inadvertently introduced and generate a positive feedback loop that exacerbates the issue. In Google’s case, their response included the creation of a team focused on AI governance to review new AI research applications for potential bias. Scientific research policies should follow suit and advocate for the extensive verification of potential bias in AI and take steps to reduce bias in databases used to train AI algorithms to ensure they are ethical and more effective.
Currently, no regulatory framework exists in Canada to modulate the use of AI and LLMs. Bill C-27, which is legislation currently under consideration within the house of commons, serves to regulate AI through the Artificial Intelligence and Data Act (AIDA). The AIDA outlines requirements for new AI models to address the intended uses and limitations of the platform, potential biases and provide relevant risk mitigation strategies. However, AIDA notes that these requirements are applicable explicitly for businesses, and no requirements are outlined for other relevant applications like scientific research. For the scientific community, the Organisation for Economic Co-operation and Development (OECD) recently released a comprehensive review of the challenges and opportunities of AI in research that could serve as a template for the development of more targeted requirements through the AIDA. More proactively, it is vital that research institutions, where a significant portion of new AI models are developed, heighten awareness, and potentially establish policies mandating comprehensive training for researchers regarding the inherent limitations of AI and LLMs. A robust approach would involve ensuring that such training is ingrained within research institutions and graduate studies, thereby fostering a foundational understanding of these intricacies.