Clinical Data Sharing and Transparency in the Time of COVID-19
Author(s):
Niamh McGuinness, PhD
Clinical Trial Transparency Team at Privacy Analytics
Senior Analyst
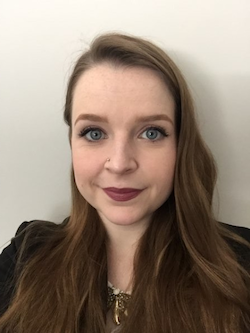
The sudden emergence of novel coronavirus COVID-19 in Wuhan, China in November 2019, resulting in a global pandemic is one of the most serious human health emergencies in recent memory. This crisis triggered a huge increase in global research efforts – the viral genome was rapidly sequenced in China (1) and RT-PCR test kits were developed in several locations worldwide by February 2020 (2). Pharmaceutical companies and academic institutions all over the world have mobilized and to date, over 960 clinical trials with a focus on vaccine or treatment development have been registered (3).
While the fear of revealing trade secrets or losing academic credit usually shrouds research in secrecy, the COVID-19 pandemic has seen unprecedented collaboration from across the scientific community (4). Robust and defensible data sharing strategies that can be implemented quickly may shape the efficacy of pandemic response efforts. Indeed, the importance of the timely exchange of clinical data was highlighted during the 2014-2015 Ebola epidemic in West Africa, resulting in a statement from the World Health Organization (WHO) to that effect in November 2015 (5).
A movement toward greater clinical trial transparency has been slowly gathering momentum for over two decades, with requirements that clinical trial sponsors provide summary and trial outcome information for studies conducted all over the world (6). More recently, initiatives like the European Medicines Agency’s Policy 0070 (7) and Health Canada’s Public Release of Clinical Information (8) have necessitated the publication of clinical documents on open-access portals. These policies have brought not only clinical trial information into the mainstream, but also the concept of anonymized participants and the importance of protecting patient privacy.
Both of the initiatives above require that patient information is anonymized such that there is reasonable assurance the information is non-identifiable (8) which can be achieved most effectively through the utilization of a quantitative, risk-based approach to anonymization. Such methods, properly applied, ensure that it is unlikely that a person can be identified, which has been demonstrated empirically through rigorous testing (9). Briefly, this strategy can be applied to ensure that patient information is transformed sufficiently to preserve privacy while maximizing data utility.
Timely anonymization of large clinical datasets such that they can be released quickly to contribute to COVID-19 research efforts is likely to prove a challenge for most data custodians. It is generally accepted that to derive as much usefulness from anonymized data as possible, a risk-based approach that considers not only the data but the release context is the optimal strategy (10,11). Realizing that expert de-identification services could be leveraged to expedite COVID-19 clinical trial data sharing, Privacy Analytics announced that they would waive fees for anonymizing pivotal and vaccine trials data (12).
In contrast to mandated publication of anonymized clinical documents under global regulations, structured clinical trial data is predominantly shared on a voluntary basis. Organizations like Vivli, who facilitate data sharing and analysis on their secure platform, are instrumental in enabling re-use of clinical data to serve the global research community and contribute to positive health outcomes. Vivli currently has 24 members and has shared data pertaining to over 5,000 clinical trials, representing some 2.7 million participants (13). To further their contribution to the global research landscape, Vivli has also announced the launch of a dedicated COVID-19 portal for sharing clinical trial data, on which all data will be hosted free of charge (14).
Privacy Analytics and Vivli are contributing their respective expertise and resources to a COVID-19 response consortium (12). Together, both organizations are committed to accelerating the anonymization and sharing of vitally important COVID-19 clinical trial data such that it can be used most effectively to combat this pandemic.
COVID-19 is likely to have far-reaching effects across the entire clinical research community which will be felt for years to come. A potential positive to come from this would be for this spirit of collaboration and voluntary sharing of data to remain entrenched in the ethos of each pharmaceutical company and academic institutions, to better meet human health challenges in the future. Privacy is not a barrier to information sharing and can in fact ensure trustworthy relationships are established between society and the research community when the appropriate protections are put in place (15).
References
1. Ren et al. (2020) Identification of a novel coronavirus causing severe pneumonia in human – a descriptive study, Chinese Medical Journal, Volume published ahead of print.
2. Sheridan, C. (2020) Coronavirus and the race to distribute reliable diagnostics, Nature Biotechnology 38, 382-384.
3. https://citeline.informa.com/trials/results?qId=6d35622d-0da5-4297-b351-50b4d4bd5731
4. https://www.who.int/news-room/detail/13-04-2020-public-statement-for-collaboration-on-covid-19-vaccine-development
5. https://www.who.int/medicines/ebola-treatment/blueprint_phe_data-share-results/en/
6. https://www.phusewiki.org/docs/Deliverables/PhUSE%20White%20Paper-%20A%20Global%20View%20-%20FINAL%20v1.0.pdf
7. European Medicines Agency. External guidance on the implementation of the European Medicines Agency policy on the publication of clinical data for medicinal products for human use. Technical report, October 2018.
8. Health Canada. Public release of clinical information: guidance document. Technical report, March 2019.
9. Branson, J. et al. (2020), Evaluating the re-identification risk of a clinical study report anonymized under EMA Policy 0070 and Health Canada Regulations, Trials, 21, 200.
10. Information and Privacy Commissioner of Ontario. De-identification guidelines for structured data, June 2016.
11. PhUSE. De-identification Standard for SDTM 3.2, 2015.
12. https://privacy-analytics.com/de-id-university/news/putting-our-passion-into-action-against-covid-19/
13. https://vivli.org/
14. https://vivli.org/vivli-covid-19-portal-2/
15. https://priv.gc.ca/en/privacy-topics/health-genetic-and-other-body-information/health-emergencies/gd_covid_202003/