Regulating AI Under Uncertainty: Challenges and Strategies
Author(s):
Piers Eaton
University of Ottawa
PhD Student
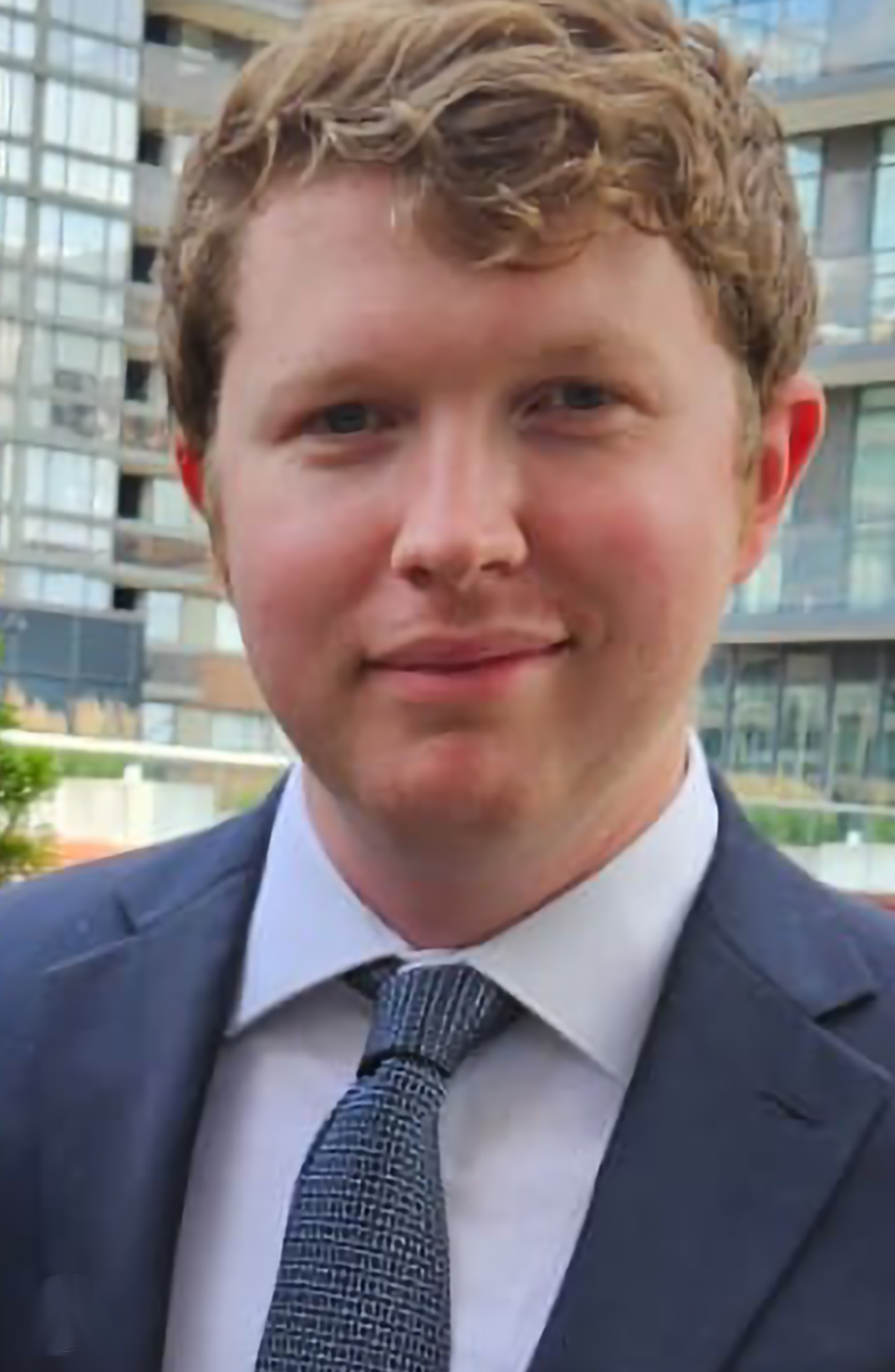
Disclaimer: The French version of this editorial has been auto-translated and has not been approved by the author.
Donald Rumsfeld is famous for, among many things, claiming that there are known knowns, known unknowns, and unknown unknowns. It was this last category, the unknown unknowns, that he identified as the type that tended to be difficult for what he saw as free countries. Unlike authoritarian countries, free countries require (often lengthy) debate before changing laws and operate on broad consensus, and most importantly constrain the power of those enforcing the law and maintaining political order.
Artificial intelligence (AI) and large language models (LLMs), of which ChatGPT is the most popular, involve all three of his variations on knowing and not knowing.
First, the known knowns. I know students use ChatGPT to do their assignments for them. I know that ChatGPT can reproduce the first line from Hamlet but struggles with the ninth (although it can get there with some guidance).
Second, the known unknowns. AI and LLMs are often black boxes, giving the public (and regulators) a limited view into their operations. We don’t know the specifics of how ChatGPT comes up with its answers. Even if it was a glass box, with a dataset as large as ChatGPT’s it is not possible to really know how an LLM is creating its answers.
Even if ChatGPT was a glass box and we could understand all its inputs, would we understand how it generates language? We hardly understand how humans have acquired language, so it seems doubtful. Many questions are left unanswered in this domain. Could ChatGPT create its own language? If it did, would it follow the universal grammar identified by Noam Chomsky? Would humans be able to understand a language produced by ChatGPT?
Other known unknowns include the rate of improvement. We can only guess when LLMs will reach particular milestones.. The point when it will impact certain job sectors is also a known unknown.
And, as Rumsfeld claimed, the unknown unknowns tend to be the most difficult. As Nick Bostrom has noted in his work Superintelligence, a sufficiently intelligent AI would use means we cannot anticipate, have goals that we did not program in it, and be capable of breaking out of our control through ways we may not see coming. Although these problems are themselves known unknowns, they suggest the possibility of extremely consequential unknown unknowns.
This reality suggests that the crafting of legislation surrounding AI and LLMs is taking place under conditions of intense uncertainty. Quite a lot of regulation is made in these kinds of circumstances. The famous ‘Section 230’ of the American Communications Decency Act of 1996, which protects websites from liability of user generated content on their websites, is necessary for the functioning of social media sites like Facebook and Twitter (now X),and really any place on the internet that allows user generated content. But the law was obviously not passed with social media in mind, as the creation of these sites came years later, and was facilitated by the trial and error of precursors that also relied on Section 230.
But the story of Section 230 shows exactly why it is so important to craft good legislation under situations of uncertainty. Section 230 has been criticized by conservatives, who believe it enables anti-conservative bias on the platforms (or alternatively, they argue that if the platforms are going to censor conservative speech, they are taking on the role of ‘publishers’ and should therefore not be granted Section 230 protections). Liberals argue that Section 230 protects sites that allow non-protected speech and enables them to shirk their responsibilities to protect their users from harmful content. While opposition to Section 230 is divided, support for the provision is united and they have the advantage of having to defend the status quo.
Because there are fewer veto points in the legislative process in Canada than the United States, it may be easier for our parliament to revise laws. At the same time, Canada, with a smaller technology sector than the US – whose Silicon Valley is a global technology hub – will have to take a firm stand and lead if it is to have a significant effect on the regulation of AI and LLMs.
There are a few ways to make legislating under uncertainty more effective that politicians can adopt. The first is to give those enforcing the legislation, such as regulators, bureaucrats and judges, sufficient leeway in how to enforce the law. Because situations that are not anticipated will arise, a lack of flexibility in enforcement can produce suboptimal outcomes. In the worst of circumstances, it can force regulators to knowingly produce bad outcomes.
The second way to legislate effectively under uncertainty is to make the goal of the legislation very clear. It’s not enough for the goal to be mentioned in parliamentary discussions and press conferences with ministers; the goal should be mentioned in the legislation itself. A clear goal will allow regulators and judges enforcing the legislation to clearly identify instances where enforcement is falling short of – or even running contrary to – the goals of the legislation.
Finally, ensure that regulators are regularly accountable to the democratic institutions from which they derive their authority. If they have to regularly come in front of parliament to explain how they are achieving the goals set out for them, then they have to explain how they are adapting to new situations and crafting new strategies. It also allows parliament an opportunity to assess if the current regulators should be replaced.
These last two legislative strategies alleviate a worry that may arise from the first strategy to reduce uncertainty – the erosion of the authority of democratic institutions through the increased leeway for non-democratic bodies. Empowering non-democratic institutions can lead to a bureaucratic state that operates to further its own ends rather than those set out by its creators, so ensuring accountability can help mitigate this concern.
There is always a degree to which legislators craft policy under uncertainty, however when it comes to anything involving AI and LLMs, both the risks and the uncertainty are greater than normal. The potential for plenty of unknown unknowns surrounding LLMs make legislation that is clear in its goals and flexible in its implementation, with accountable regulators, essential for effective regulation.