Speed versus Truth: Conferring legitimacy on an expedient system
Author(s):
Dean Shamess
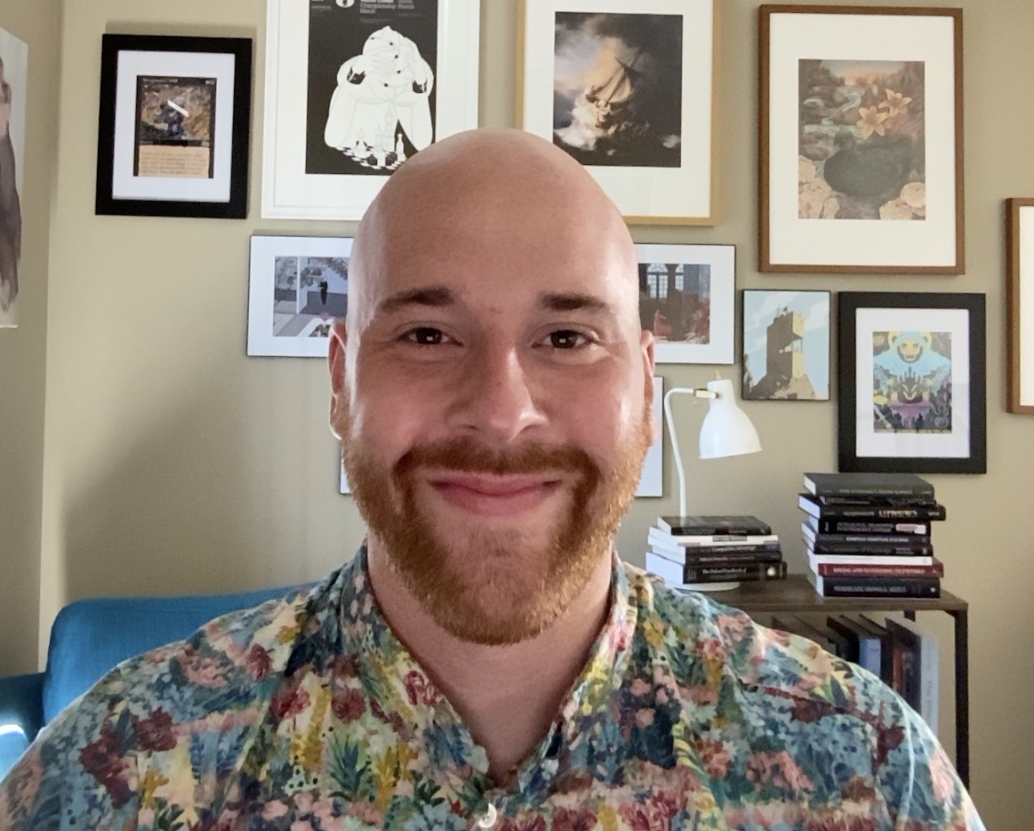
Disclaimer: The French version of this editorial has been auto-translated and has not been approved by the author.
I have absolutely no material stake in the success or failure of any given AI system or company, at least no more or less than any other person with no monetary investments in them.
I’m also not the first or only person to suggest that AI, and large language models (LLMs) more specifically, may not be existential risks in the sense of our worst science fiction nightmares. But, unlike those nightmares, I do think there are AI advances that may spell something akin to a social extinction. However, even that prediction can be tempered through reasonable regulation and governance, if we understand the fundamental things that need regulating.
Let’s start here: innovation is getting harder. Evidence and anecdote about this argument is everywhere but I’m going to premise this point on Jones’ 2009 article in the Review of Economic Studies, ‘The Burden of Knowledge’ (https://www.jstor.org/stable/20185091). New inventors face an increasing educational burden as each prior generation has pushed the knowledge frontier further and further out. This is why, to some extent, academic and industrial scientists have increasingly specialized – it reduces the burden.
One of the potential solutions to this burden offered by Jones is that there could be a future technology that makes knowledge diffusion/acquisition efficient enough that the cost to reach the knowledge frontier is lessened. AI and LLMs seem like exactly this type of technology – and that’s great!
But for an LLM to make the process of diffusion and acquisition better, it would have to beat the status-quo – the internet as it exists today. Right now, I can find credible, insightful, thoughtful information on the internet with relative ease without the use of a generative AI. However, I still must spend significant time thinking critically about the results I’m given by a search engine:I need to sift through articles to find what is most or least related to what I actually care about and, I have to decide afterwards what I think should actually matter in how I view the topic at hand. So how could an LLM make this better? The obvious answer is that it can synthesize and distill lots of information in less space and require less of my time to reach understanding. That’s also, maybe, great!
The cost of this is that we start getting answers to important questions that “sound like what an answer should sound like, which is different from what an answer should be” (https://spectrum.ieee.org/gpt-4-calm-down). As generative AI improves, we’re likely to confer more and more authority to it and, de facto, to its creators. When that happens, we’ve conferred authority onto a system that, by necessity, has reduced complexity into simplicity.
We make ourselves subject to the generative AI process, and give it authority over much of truth, by trading time and effort for simplicity.
This leads us to my criticism of and urging for good governance of AI and LLMs. Sam Altman, CEO of OpenAI and the ostensible leader in market-shaping, generative AI right now, argued for AI regulation to the United States Senate recently. He should be applauded for this, but we cannot ignore his enormous stake in the success of OpenAI – if regulatory walls are built such that the burden of competition is too great, then we have conferred authority onto him and his FAANG colleagues.
This is the first and maybe most obvious tradeoff that policymakers must consider when approaching AI regulation. How do we conceive of a barrier to entry that is sufficiently low to allow for entry and competition (i.e.: to not solely legitimate and facilitate the biggest tech co.’s from monopolizing this space) while also regulating against a potentially socially corrosive product?
If we imagine a world where generative AI has “expertise” and the conferred authority of reliably summoning that expertise to explain the world to us, that relieves some of the burden of knowledge. But if the systems, or the people building the systems, that explain the world to us do so in a way that feeds into our need for simplicity, we lose the complexity and richness of the world that gives us truth.
When I asked ChatGPT how we should regulate LLMs so that their social benefits are maximized while their costs are minimized, I was offered more than ten potential ideas:
“To regulate large language models (LLMs) effectively, consider transparency, accountability, data privacy, bias detection, quality assurance, algorithmic auditing, collaboration, ethical guidelines, monitoring, education, and international cooperation. This approach aims to maximize benefits while minimizing risks.”
ChatGPT was also readily able to expand on each of these ideas, providing a little more nuance and depth to what are, frankly, ideas so broad as to be unusable. This seems fine to me! But, in a world where LLMs are significantly more powerful than what we have today, and where we confer more and more authority onto them, they provide yet another window for us to wall ourselves off from one another. We lose complexity and messiness when we seek the simple and should be wary of that simplicity … that lack of truth.
Regulation of AI and LLMs should be focused on preventing a future where we forego the truth in favour of the most expedient approximation of it. I don’t believe that we’re headed towards a world where humans are destroyed at the hands of a sentient AI, but we might be headed towards one where we destroy the connections we share and the richness of our incredibly complex world.